One very common logical fallacy I see in the US is the confusion of correlation with causation. Take the book Codes: The Making of a New Tribe and Remaking of the World, in the very first chapter the authors ridiculously fall flat faced on this fallacy. They claim that they have data to prove their conclusions which ended up being: Coders are sexist and racist as a general rule along with the companies who hire them.
The logic between this was simply made by looking at the demographics and concluding that if it doesn’t reflect the general population it must therefore be a sign of a bias. So since coders are mostly men, there must be a bias against women. Since coders are mostly of European and Asian ancestry they must be racist to other groups. The authors of this book made some serious non sequiturs hardly anyone who’s read it has pointed it out.
First of all coders are made of individuals that made a career choice, and this choice doesn’t affect those of others. You can’t say that as a general rule coders are sexist and racist if the people choosing this career choice as a whole don’t reflect the general population. This is like saying elementary school teachers are generally sexist since they’re mostly woman who pick this career and this doesn’t reflect the general population, a statement most people would agree is false while accepting that coders are sexist. The same applies with race.
Secondly, to say that companies are sexist and racist because their coders don’t reflect the demographics of the general population is equally ludicrous. The book states that since companies don’t have a 50/50 hiring ration between men and women they are therefore engaging in sex bias when hiring. This logic is ignoring that the pool of candidates are already overwhelmingly male, and unless you’re going to discriminate against men and have about eight or nine unemployed male coders for every hired male while almost a full employment for women you’re not going to have an even split (not to mention that this last scenario would be the bias they’re accusing the industry of engaging).
This simple arithmetic seemed to have eluded the authors. You have an industry with relatively low unemployment and dominated by male applicants, how the hell do you claim the hired workforce should be 50/50 or there’s a sex bias?
One of the funniest conclusions of this first chapter is to say smaller companies are even more bias than large companies. The logic for this was to say that the sex ratio is closer to 50/50 in large companies, and the gap is larger in smaller. Well, if large companies are hiring women at a higher rate than those available in the general pool of candidates then the smaller companies by default are left with a higher male vs female ratio. The authors again fail to address basic arithmetic.
Another of the issues I’ve seen addressed with specifically with the bias of Data Scientists and machine learning. This topic has appeared in LinkedIn and many articles. The basic logic is this: More men get loan approvals than woman therefore the algorithms must have a bias against women and must be corrected to reflect a 50/50 sex ratio of approval and the Data Scientists are accused of sexism. Similar claims to racial bias are made, but which they conveniently leave out the dozen or so nationalities that have a higher approval rate than the whites they claim are being favored by said bias.
The problem with these criticisms is that they claim the credit process has different rules for different groups (credit score, income, debt, etc applied differently) without logically proving that these rules aren’t equally being applied and groups perform different. They simply look at a correlation (race, sex) and if there’s a difference in outcome claim this is a sign of bias that needs to be corrected, but ironically the very correction would be the bias they claim to fight since it would have to apply different approval rates (credit score, income, debt, etc) based on the sex and race of the individual and not equally applied regardless of these factors.
Not to mention that a common one that I heard is that the results don’t match their desires because the programmer must have been a “white male” applying biases. This Ad Hominem creates the very same bias against sex and race they falsely claim to be fighting against they they spit out their logical fallacies. But seems like their cognitive dissonance can be endless.
In this data set I’ll analyze Credit Approval Data Set ( https://archive.ics.uci.edu/ml/datasets/Credit+Approval ) and separate the correlation (higher number of males approved) with the causes (income, debt, credit score and previous default) to find out if there’s a bias in favor of men in credit approval as I’ve so often heard in the past year.
The following graph shows you the approved count at 307 and rejected count at 383 for the data as a whole:
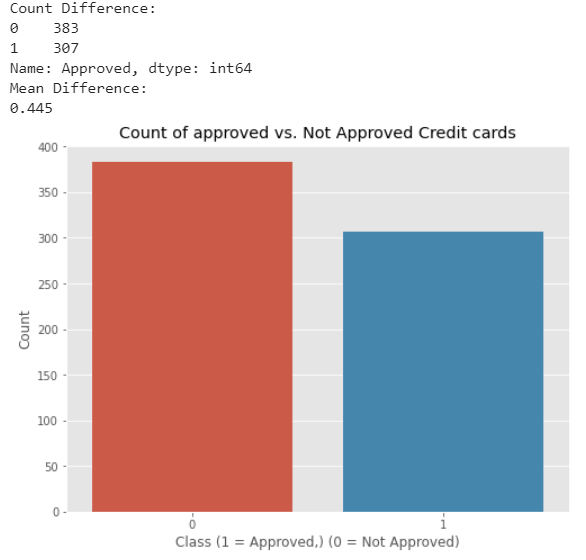
The following two graphs shows the approval and rejection of males and females, overall more men are rejected based on the number of applicants than women:
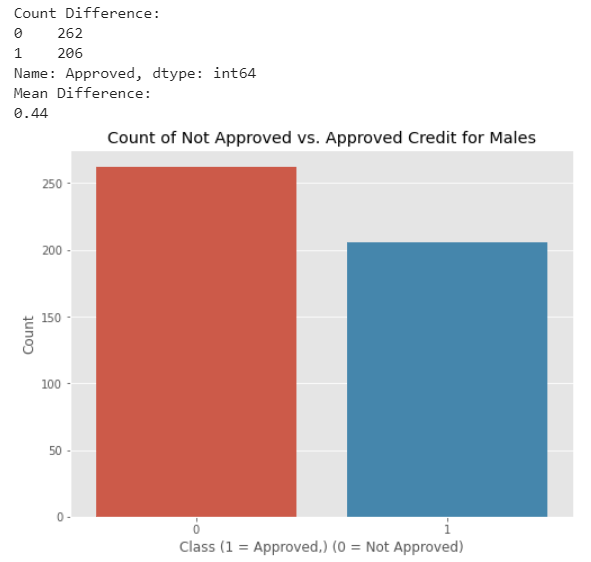
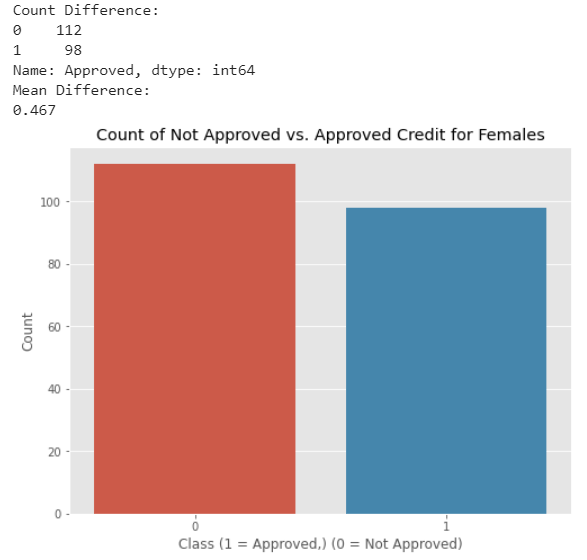
The confused crusaders would be quick to say that since more men get approved than women there must be a a bias in favor of men, but looking at the data as a whole there were far more men who applied, and more men were rejected vs approved than that of women:
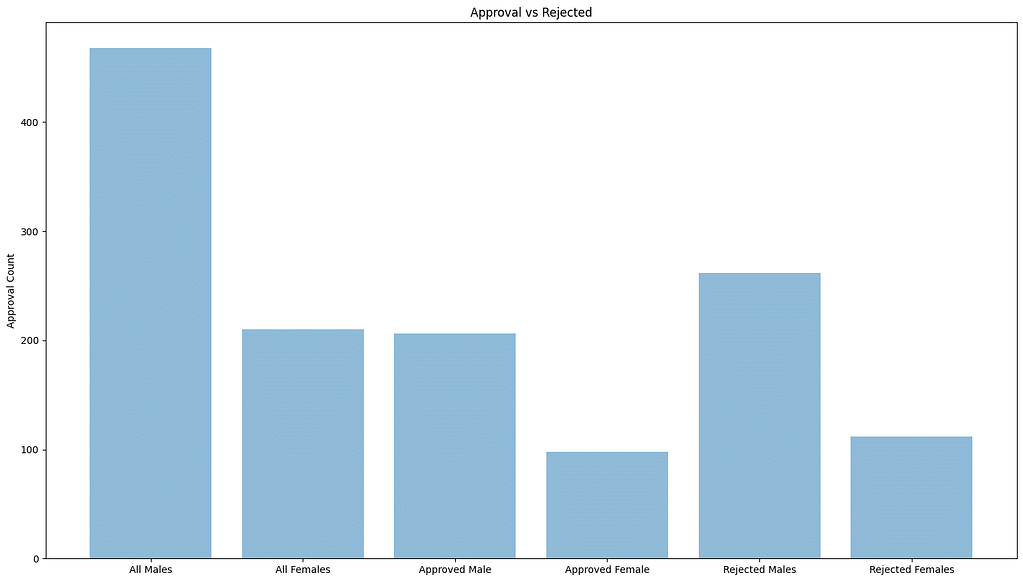
The following summary is based on the averages of the data and the main causalities of Prior Default, Debt, Credit Score, and Income:
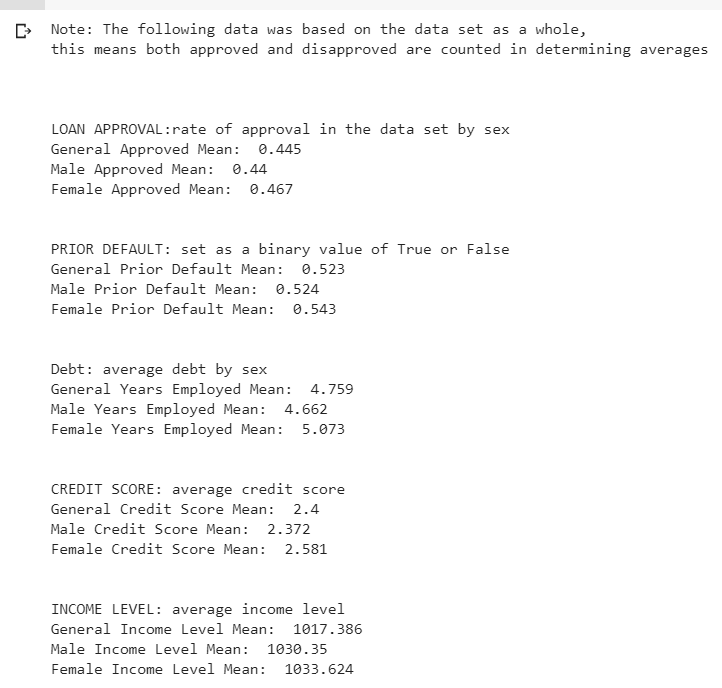
The numbers seem relatively similar, now I’ll separate the data with approved and disapproved applicants.
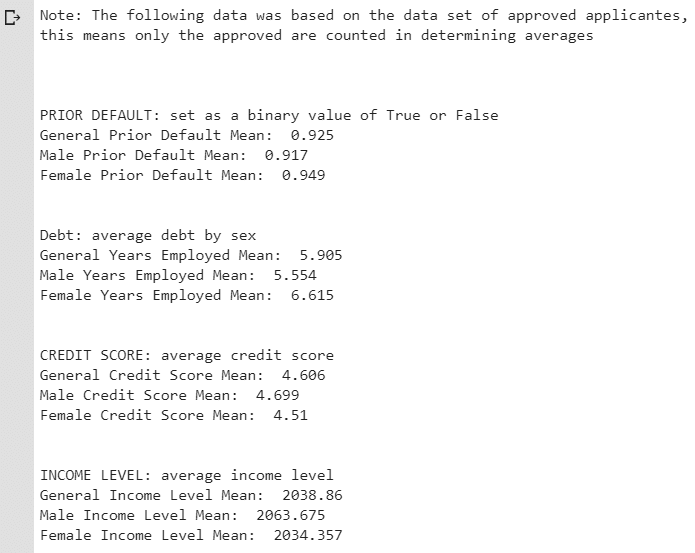
Both groups in the approved category seem relatively equal in income and credit scores, but Men had significantly less debt and somewhat less previous defaults.
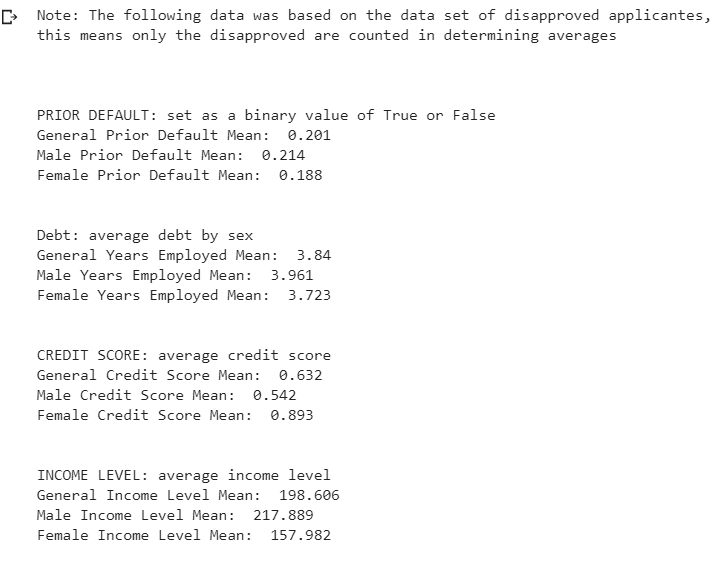
Summary of the data:
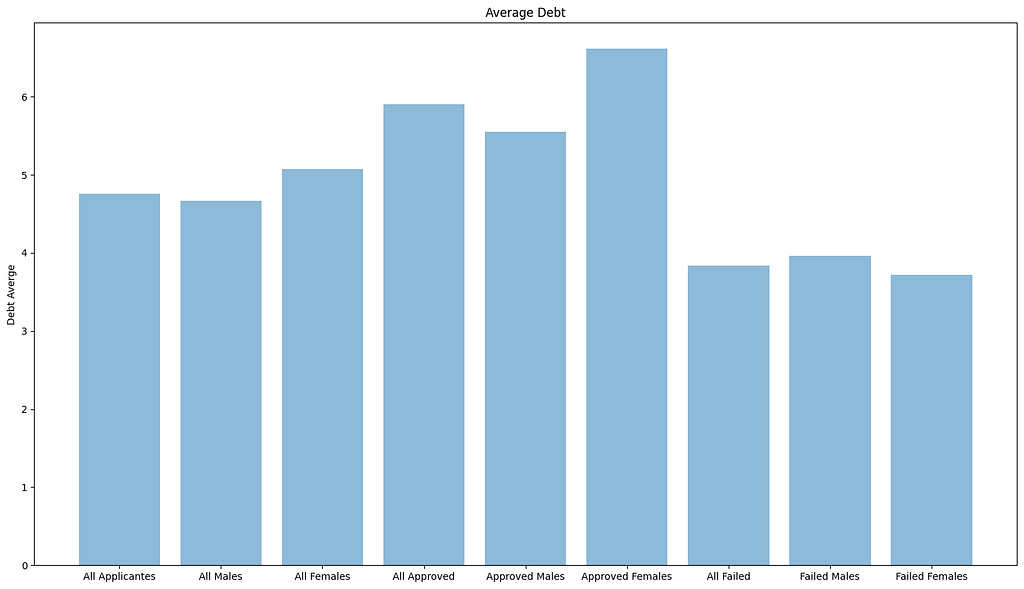
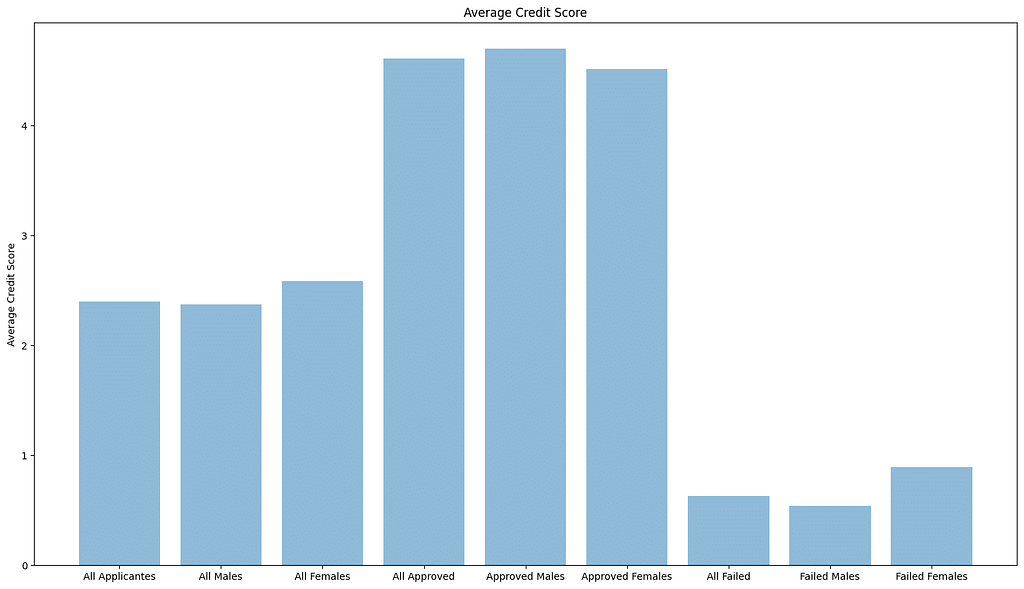
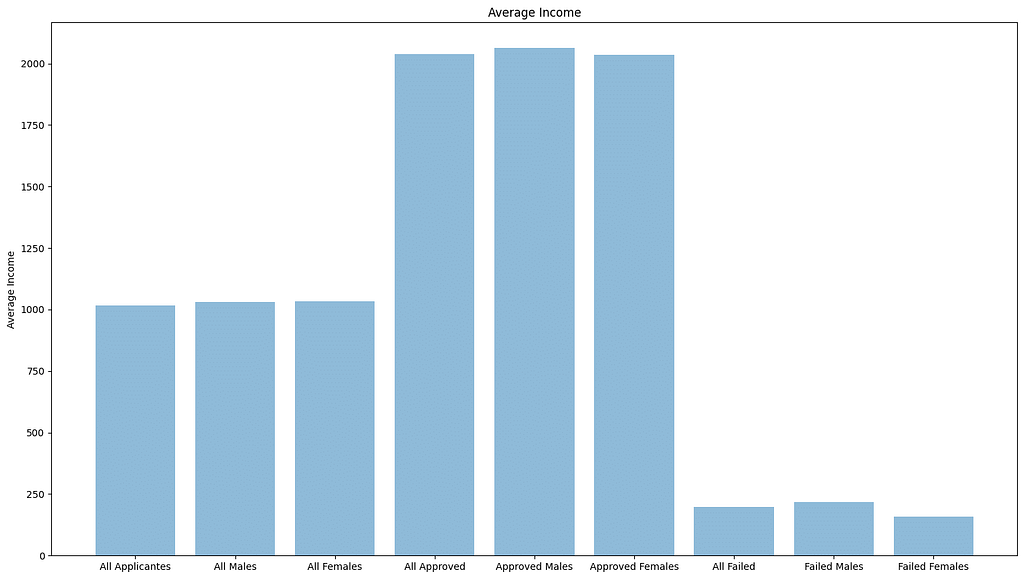
Looking at the data as a whole seems that the causalities are prior default, debt, credit score and income. The men and women that got approved score relatively similar in average to each other, as do the men and women who got rejected. Having had more men approved is simply a matter of having had more men who qualified with these mentioned factors applied and there isn’t a bias in the approval process.
You’re so awesome! I do not suppose I’ve read a single thing like this before.
So nice to discover another person with a few unique thoughts on this issue.
Seriously.. many thanks for starting this up.
This website is something that’s needed on the web, someone with some originality!